Kerr (2013) recently provided a critical review of regional climate models (“RCMs”). I think his views have caused a stir in the regional climate model community. So what’s the buzz all about?
RCMs provide important input to many climate services, for which there is a great deal of vested interest on all levels. On the international stage, high-level talks lead to the establishment of a Global Framework for Climate Services (GFCS) during the World Climate Conference 3 (WCC3) in Geneva 2009.
Other activities include CORDEX, and the International Conference on Climate Services 2 (ICCS2). On a more regional multi-national level, there are several activities on climate services which have just started, and, looking only in Europe, there are several big projects: JPI-Climate, SPECS, EUPORIAS, IMPACT2C, ECLISE, CLIM-RUN, IN-ENES, BALTEX, and ENSEMBLES. Many of these projects rely on global and regional climate models.
There are well-known limitations to both global and regional climate models, and many of these are described in Maslin and Austin (2012) as “uncertainty”. Maslin and Austin highlighted several reasons for why the regional-scale predictions made by these models are only tentative, and Racherla et al. (2012) observed that:
there is not a strong relationship between skill in capturing climatological means and skill in capturing climate change.
They acknowledged that the problem is not so much the RCMs, but the global climate models’ (GCMs) ability to predict climate changes on a regional scale. This finding is not surprising, however, it is important to establish this fact for the record.
Racherla et al. assessed the skill of an RCM and a GCMs, based on simulated and observed temperature and precipitation for two 10-year time slices (December 1968-December 1978 and December 1995 – December 2005). While they realised that estimating change from two different ten-year intervals is prone to errors caused by spontaneous natural year-to-year (and even slower undulations in temperature and precipitation – e.g. the AMO), they argued that such set-up was common in many climate change studies.
This aspect takes us back to our previous post about the role of large-scale atmospheric circulation associated with natural and ‘internal’ variations. The GCMs may in fact be able to reproduce many of the year-to-year variations and the slower variations, however, we know that these fluctuations are not synchronised with the real world.
The apparent lack of skill may not necessarily be a shortcoming of the individual climate models – indeed, they successfully compute the sensitivity of the subsequent large-scale atmospheric flow to small differences in their starting point.
These variations come on top of the historical long-term climate change trend. In the past, the regional natural variations have often been more pronounced than the regional climate change, and if they are out of synch, then we should expect neither a RCM nor a GCM to be able to predict the change between the two decades.
Hence, the fact that the past has been blurred by natural year-to-year variations does not invalidate the climate models. A proper evaluation of skill would involve looking at longer time scales or many different model runs. One important message is that one should never use a single GCM for making future regional climate projections.
For proper validation, we must look at a large number of different simulations with GCMs, and then apply a statistical test to see if the observed changes are outside the range of changes predicted by the models. By running many models, we get a statistical sample of natural variations following different courses.
Running RCMs is computationally expensive and it may not be possible to let them compute results for many decades or many GCMs. However, empirical-statistical downscaling (ESD) is an alternative that does not require much computing power. ESD and RCMs have different strengths and weaknesses, and thus complement each other.
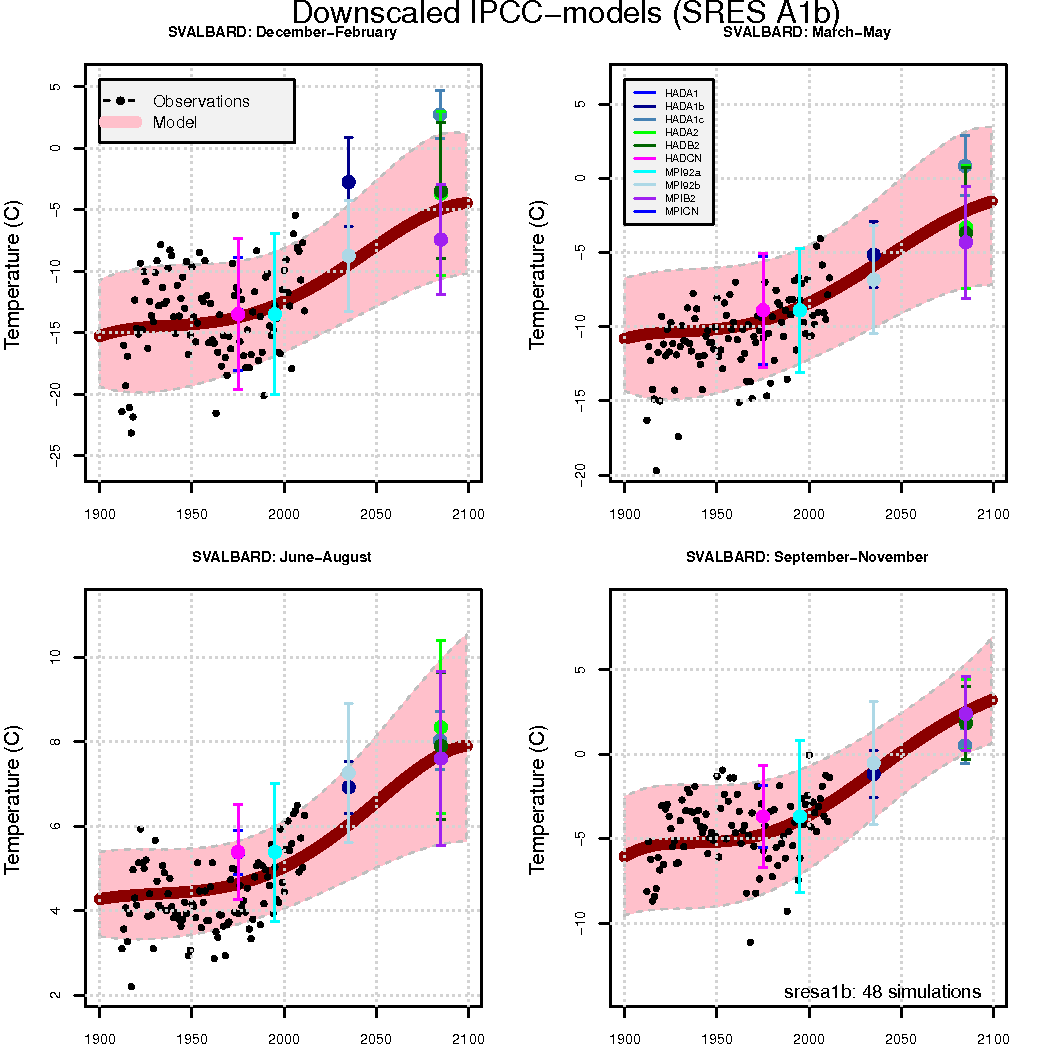
The figure above, taken from Førland et al. (2012) shows a comparison between ESD and RCM results for the Arctic island Spitsbergen (a part of the Svalbard archipelago), where the ESD has been applied to the entire 1900-2100 period as well as 48 different GCM simulations.
Racherla et al. (2012) also discussed another concern, which is how RCMs and GCMs are combined. Since RCM only cover a limited space, the values at their boundaries must be specified explicitly (referred to as ‘boundary conditions‘), by the results from a coarser GCM or observation-based data (reanalysis).
The GCMs used to force the RCMs, however, do not account for situations where they and the RCMs describe a different states (e.g. precipitation or wind). This problem arises in the situation called upscaling, where small features grow in spatial extent (not atypical for chaotic systems).
It is possible to remedy some of the inconsistencies between the large-scale flow in the RCMs and the embedding GCMs by imposing so-called ‘nudging’.
Furthermore, imposing boundary values on models like RCMs may also sometimes cause problems such as spurious oscillations, and are by some labelled as an “ill-posed problem“. These problems can nevertheless be alleviated by using a “buffer-zone” along the RCM’s boundaries.
A finer grid mesh in the RCMs gives an improved description of mountains over that in the GCM, and introduces further details sugh as higher mountain peaks. This improvement alters the way air is forced upward over mountains, compared to the coarser GCM, and the amount precipitated out (‘orographic precipitation’).
Different ways of computing the cloud processes (cloud parameterisation) affect the condensation of vapour, the outgoing long-wave radiation, and precipitation.
A finer spatial grid also affects the wind structure and the evaporation near the surface (which depends on the wind speed). Furthermore, the energy transported in the atmosphere through eddies may not correspond between models with fine and coarse resolutions respectively.
Such differences between RCMs and GCMs may lead to inconsistent physics, however, are these concerns important, or just second-order effects?
Once again, a comparison between ESD and RCM results will provide some idea, and in many cases, there is a fair degree of agreement between these downscaling strategies. The problems with RCMs are absent in ESD (which have different caveats), however, the important question is whether the GCMs, used to drive both, provide a realistic description of the regional climate.
The figure above indicates that the GCMs (and the ESD results) underestimate some of the local natural variations in the past – which probably are connected with the Arctic sea ice (Benestad et al., 2002). The GCMs used in these calculations do not seem to capture the recent decline in the Arctic sea-ice cover (Stroeve et al., 2012).
Another problem may be that the RCMs do not represent the precipitation statistics well, even when data based on real observations (the ERA40 reanalysis) are used to provide the boundary values (Orskaug et al., 2011). For climate services, it is important that the precipitation statistics is realistic, and in the past, systematic biases have been “fixed” (in a not very satisfactory way) by bias-correction.
Boberg and Christensen (2012) presented one type of validation analysis, that may partially meet the concerns expressed in Kerr (2013). They reported that many RCMs overestimate the temperature in warm and dry climates (e.g. around the Mediterranean). This temperature bias was greater with higher temperature.
Most of the GCMs too had similar temperature biases, suggesting that the deficiencies seen in the RCM results were not different to those in the GCMs. Furthermore, these deficiencies cannot be explained in terms of the differences between the measured temperatures and the data used as boundary conditions for the RCMs (see figure below).
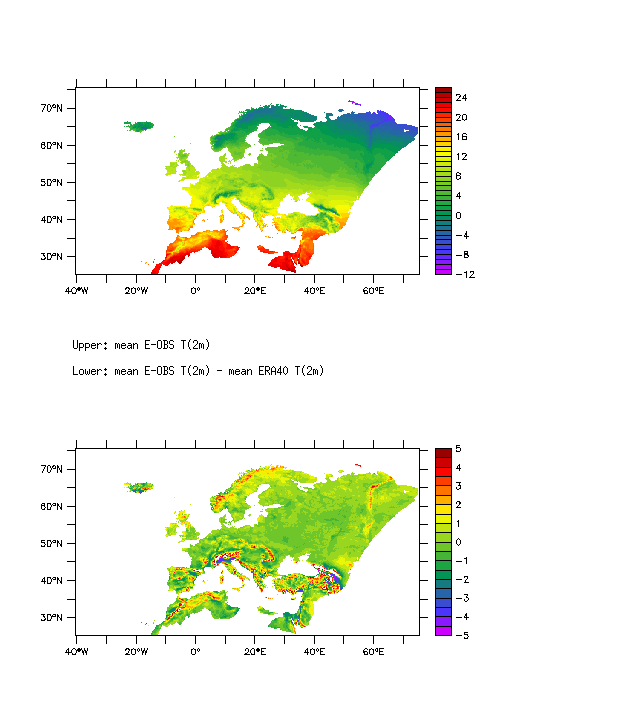
According to Kerr (2013), the RCMs need a more thorough validation, addressing the question whether they are able to describe changes in the local climate.
It is also important to verify that they actually provide a consistent representation of the physics when embedded in the GCMs. Do the energy and mass (moisture) fluxes across the lateral and top boundaries of the RCM correspond with the fluxes through the cross-sections in the GCMs corresponding to the RCM’s boundaries?
There are also new initiatives on proper validation of regional climate modelling (ESD and RCMs), and the European project VALUE represents one notable example.
(p.s. One of the references below has wrong author and title, but correct link and DOI).
References
- R.A. Kerr, "Forecasting Regional Climate Change Flunks Its First Test", Science, vol. 339, pp. 638-638, 2013. http://dx.doi.org/10.1126/science.339.6120.638
- M. Maslin, and P. Austin, "Climate models at their limit?", Nature, vol. 486, pp. 183-184, 2012. http://dx.doi.org/10.1038/486183a
- P.N. Racherla, D.T. Shindell, and G.S. Faluvegi, "The added value to global model projections of climate change by dynamical downscaling: A case study over the continental U.S. using the GISS‐ModelE2 and WRF models", Journal of Geophysical Research: Atmospheres, vol. 117, 2012. http://dx.doi.org/10.1029/2012JD018091
- E.J. Førland, R. Benestad, I. Hanssen-Bauer, J.E. Haugen, and T.E. Skaugen, "Temperature and Precipitation Development at Svalbard 1900–2100", Advances in Meteorology, vol. 2011, pp. 1-14, 2011. http://dx.doi.org/10.1155/2011/893790
- R.E. Benestad, E.J. Førland, and I. Hanssen‐Bauer, "Empirically downscaled temperature scenarios for Svalbard", Atmospheric Science Letters, vol. 3, pp. 71-93, 2002. http://dx.doi.org/10.1006/asle.2002.0050
- J.C. Stroeve, V. Kattsov, A. Barrett, M. Serreze, T. Pavlova, M. Holland, and W.N. Meier, "Trends in Arctic sea ice extent from CMIP5, CMIP3 and observations", Geophysical Research Letters, vol. 39, 2012. http://dx.doi.org/10.1029/2012GL052676
- E. Orskaug, I. Scheel, A. Frigessi, P. Guttorp, J.E. Haugen, O.E. Tveito, and O. Haug, "Evaluation of a dynamic downscaling of precipitation over the Norwegian mainland", Tellus A: Dynamic Meteorology and Oceanography, vol. 63, pp. 746, 2011. http://dx.doi.org/10.1111/j.1600-0870.2011.00525.x
- F. Boberg, and J.H. Christensen, "Overestimation of Mediterranean summer temperature projections due to model deficiencies", Nature Climate Change, vol. 2, pp. 433-436, 2012. http://dx.doi.org/10.1038/NCLIMATE1454
Thanks – this is very helpful for those of us who use (or are thinking about using) regional model projections in policy analysis!
Thanks for the informative post.
The links to the other sites that explained the terms being used were important and useful for me. I use Real Climate to stay informed, but sometimes I find some of the concepts hard to wrap my head around. Some background and definitions make it much easier for me to understand, like the Wikipedia page on ‘boundary conditions’ and the World Scientific page on empirical-statistical downscaling.
I thought the line “So what’s the fuzz all about?” in the first paragraph was a misinterpretation by a non-native english speaker of the expression “what’s the buzz all about” until I read the link to Kerr 2013!
Thank you for this article. Just a minor grammatical point: in the sentence that begins “ESD and RCMs have different strengths” you might want to replace “compliment” with “complement.”
Eli was talking with a friend, a regional modeler on Friday, about air pollution models. He was grousing how the dumbest models (basically persistence) do the best job if nothing changes. On the other hand they fail completely if something changes (for example the ozone model used in LA failed completely during the Olympics because there was much less traffic).
When something changes the complex chemical models do a much better, tho not perfect job, of capturing the change. There may be something similar going on here, where a combination of downscaling and persistence may be needed.
Is anybody counting the plankton in this regard? The species mix can change fast and I’d imagine it would be doing so as the ice changes, and in no simple way.
> ?
belatedly taking my own advice …
https://www.google.com/search?q=regional+climate+model+plankton
Lots
I’m curious as to Rasmus & Raypierre’s take on Lovejoy & Schertzer’s new book The Weather and Climate: Emergent Laws and Multifractal Cascades. which avers that many of the shortcomings of regional and global prediction can be overcome by multifractal cascade models.
The blurb claims that :
” By generalizing the classical turbulence laws, emergent higher-level laws of atmospheric dynamics are obtained and are empirically validated over time-scales of seconds to decades and length-scales of millimetres to the size of the planet. In generalizing the notion of scale, atmospheric complexity is reduced to a manageable scale-invariant hierarchy of processes, thus providing a new perspective for modelling and understanding the atmosphere.”
Would that it were so, but…
I guess my questions are: How are RCMs constructed? Are they the result of applying finer horizontal and vertical grids to GCMs? Are there differences in the underlying physics — GCM vs. RCM? What are the principal differences between projection from RCMs versus those using ESD? What is downscaled in ESD? Whence comes the data to initialize RCMs? ESD models? Are the data the same as those used in GCMs?
At first glance, it is no small wonder “that the problem is not so much the RCMs, but the global climate models’ (GCMs) ability to predict climate changes on a regional scale.”
The interesting post points towards an issue we spend a lot of time discussing during my climate change course at UBC: the gap between what science can deliver and what people expect science to deliver. Climate services are potentially very valuable, however, we need to understand and appreciate this gap, or scientists and decision-makers will continue to frustrate one another.
As a test of the regional models, it might be interesting to concentrate on outliers – areas where the models predict unusually large climate changes, and areas where in fact changes are large. To what extent do they match? Concentrating on outliers mitigates the problem that the models cannot cover all forces because large effects are likely to drown out the background noise. Also, of course, big changes have more practical importance.
Well, that is not that much connected to the topic of this post, but I’ll ask anyway:
Regarding the IPCC-models and positive feedbacks, such as the melting of permafrost, why are such feedbacks not included in the latest report? Seems to me that they are rather too important to neglect.
> the IPCC-models
The IPCC doesn’t have any models.
You’re reading the last IPCC Report?
note the dates.
What if we take a break for let say 10 years and see what will happen. In the mean time put all our effort in what we know and do something about it with what we know.
If there is a hole in the dike the best thing to do is put your finger in it. Maybe over simplified but a lot easier than to figure out a way to lower the water level of the sea.
The world is getting sick of spending billions of dollars towards climate change research wile hardly anything is happening what is measurable. Yes we invented, solar panels, wind-turbines, electric cars, what did it bring except burning tons of energy to produce them.
I don’t say the work of scientist is not valuable, it is however you don’t save the world with data, we save the world with action.
10 years, 10 billion trees, 10 million sustainable jobs. You don’t need science to figure-out the value of that.
Piet,
Well, thankfully, scientists know far, far more than you do. We know CO2 is a greenhouse gas–and have known this since the 1850s. We know adding CO2 to the atmosphere will warm the planet. And we know your silly, little mitigation suggestions won’t matter worth a fart in a hurricane.
Might I suggest that if you start to realize just how ignorant your opinion is, it might go a long way toward rectifying your ignorance.
> afforestation
People are very good at pushing systems’ levers, but generally push them in the wrong direction. You have an example there.
Problems with the loggers’ notion that rushing to plant more trees is useful: it’s mostly wrong because trees aren’t most of the answer. Forest holds carbon where it’s left undisturbed. Mature complicated forests are pretty tolerant of fire, left alone — the fire burns through under shaded canopy between big trees, clearing out brush. Forest is mostly soil, with a top layer of trees. The problem with the timber industry approach is, you can’t get that soil back quickly, nor preserve it, when you cut the trees. Nor is most of the actual timber preserved by logging; most goes to sawdust, or rots quickly.
Most of a standing tree is dead wood, surrounded by a thin layer of living tree. Once the tree dies the dead center is rapidly converted to living fungi — both above and below ground; the dead tree is rapidly converted to — mostly living soil.
https://www.sciencemag.org/content/339/6127/1615.short
Science 29 March 2013:
Vol. 339 no. 6127 pp. 1615-1618
DOI: 10.1126/science.1231923
“50 to 70% of stored carbon in a chronosequence of boreal forested islands derives from roots and root-associated microorganisms. Fungal biomarkers indicate impaired degradation and preservation of fungal residues in late successional forests.”
> solar panels … what did it produce …?
http://news.stanford.edu/news/2013/april/pv-net-energy-040213.html
But this is going way off topic. You can look this stuff up easily.