Following on from the ‘interesting’ House Science Committee hearing two weeks ago, there was an excellent rebuttal curated by ClimateFeedback of the unsupported and often-times misleading claims from the majority witnesses. In response, Judy Curry has (yet again) declared herself unconvinced by the evidence for a dominant role for human forcing of recent climate changes. And as before she fails to give any quantitative argument to support her contention that human drivers are not the dominant cause of recent trends.
Her reasoning consists of a small number of plausible sounding, but ultimately unconvincing issues that are nonetheless worth diving into. She summarizes her claims in the following comment:
… They use models that are tuned to the period of interest, which should disqualify them from be used in attribution study for the same period (circular reasoning, and all that). The attribution studies fail to account for the large multi-decadal (and longer) oscillations in the ocean, which have been estimated to account for 20% to 40% to 50% to 100% of the recent warming. The models fail to account for solar indirect effects that have been hypothesized to be important. And finally, the CMIP5 climate models used values of aerosol forcing that are now thought to be far too large.
These claims are either wrong or simply don’t have the implications she claims. Let’s go through them one more time.
1) Models are NOT tuned [for the late 20th C/21st C warming] and using them for attribution is NOT circular reasoning.
Curry’s claim is wrong on at least two levels. The “models used” (otherwise known as the CMIP5 ensemble) were *not* tuned for consistency for the period of interest (the 1950-2010 trend is what was highlighted in the IPCC reports, about 0.8ºC warming) and the evidence is obvious from the fact that the trends in the individual model simulations over this period go from 0.35 to 1.29ºC! (or 0.84±0.45ºC (95% envelope)).
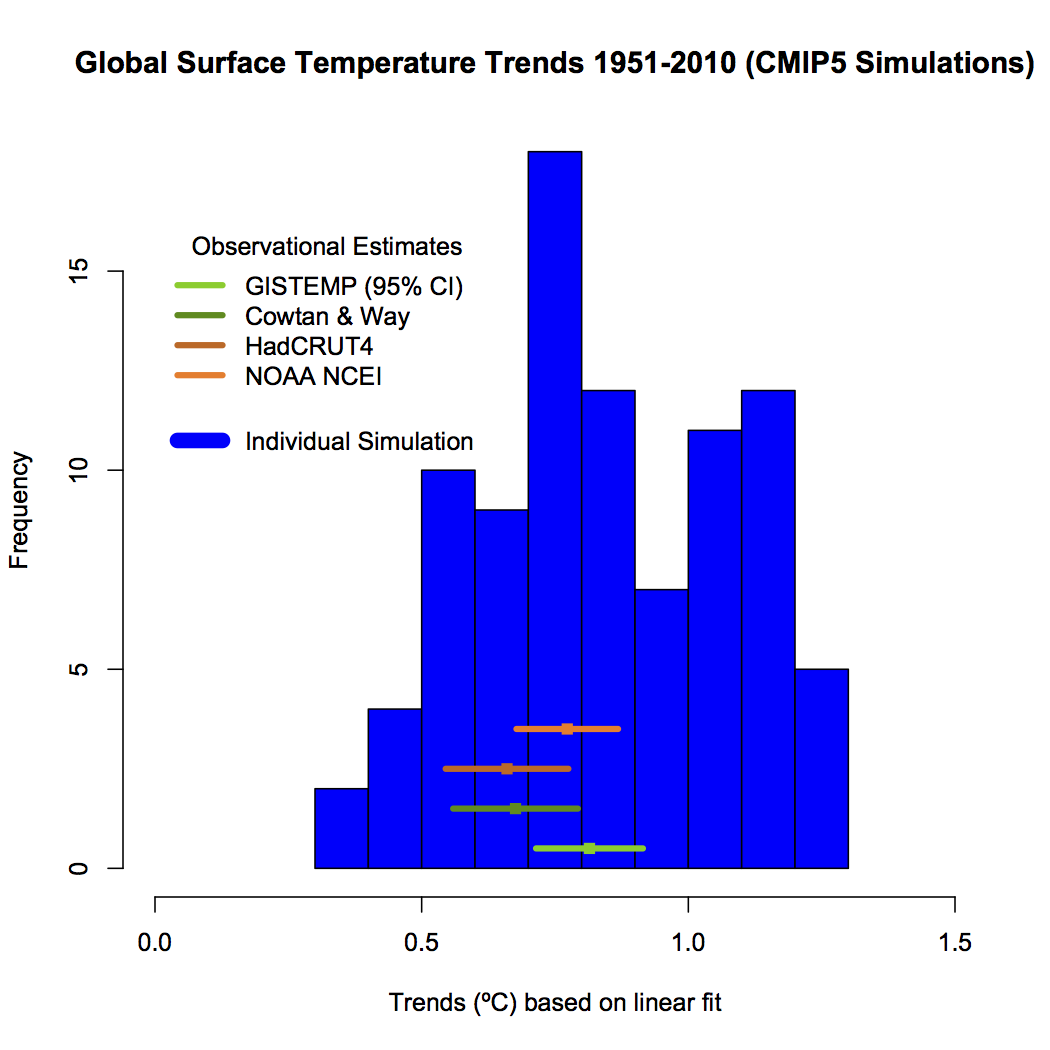
Ask yourself one question: Were these models tuned to the observed values?
Second, this is not how the attribution is done in any case. What actually happens is that the fingerprint of different forcings are calculated independently of the historical runs (using subsets of the drivers) and then matched to the observations using scalings for the patterns generated. Scaling factors near 1 imply that the models’ expected fingerprints fit reasonably well to the observations. If the models are too sensitive or not enough, that will come out in the factors, since the patterns themselves are reasonably robust. So models that have half the observed trend, or twice as much, can still help determine the pattern of change associated with the drivers. The attribution to the driver is based on the best fits of that pattern and others, not on the mean or trend in the historical runs.
2) Attribution studies DO account for low-frequency internal variability
Patterns of variability that don’t match the predicted fingerprints from the examined drivers (the ‘residuals’) can be large – especially on short-time scales, and look in most cases like the modes of internal variability that we’ve been used to; ENSO/PDO, the North Atlantic multidecadal oscillation etc. But the crucial thing is that these residuals have small trends compared to the trends from the external drivers. We can also put these modes directly into the analysis with little overall difference to the results.
3) No credible study has suggested that ocean oscillations can account for the long-term trends
The key observation here is the increase in ocean heat content over the last half century (the figure below shows three estimates of the changes since 1955). This absolutely means that more energy has been coming into the system than leaving.
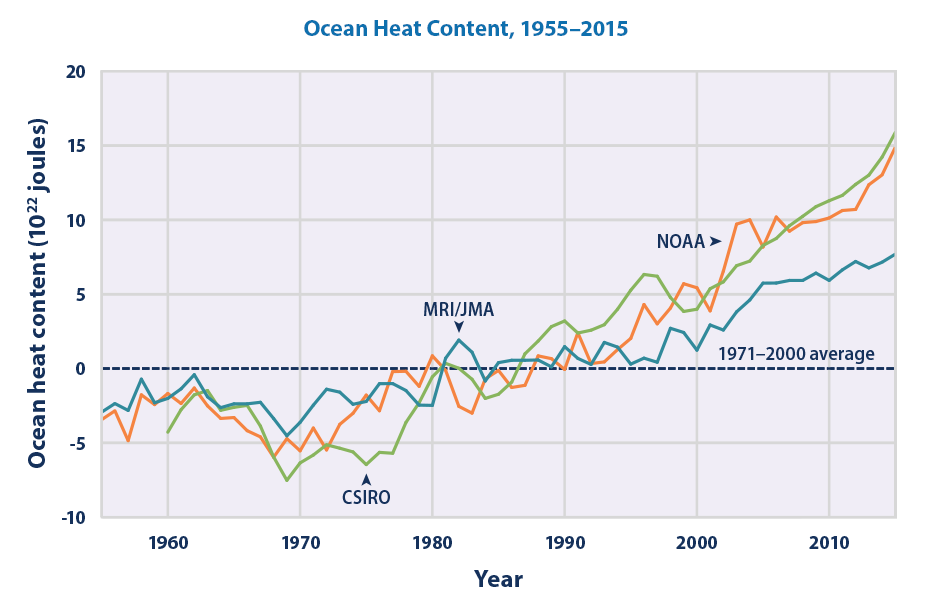
Now this presents a real problem for claims that ocean variability is the main driver. To see why, note that ocean dynamics changes only move energy around – to warm somewhere, they have to cool somewhere else. So posit an initial dynamic change of ocean circulation that warms the surface (and cools below or in other regions). To bring more energy into the system, that surface warming would have to cause the top-of-the-atmosphere radiation balance to change positively, but that would add to warming, amplifying the initial perturbation and leading to a runaway instability. There are really good reasons to think this is unphysical.
Remember too that ocean heat content increases were a predicted consequence of GHG-driven warming well before the ocean data was clear enough to demonstrate it.
4) Indirect effects of solar forcing cannot explain recent trends
Solar activity impacts on climate are a fascinating topic, and encompass direct radiative processes, indirect effects via atmospheric chemistry and (potentially) aerosol formation effects. Much work is being done on improving the realism of such effects – particularly through ozone chemistry (which enhances the signal), and aerosol pathways (which don’t appear to have much of a global effect i.e. Dunne et al. (2016)). However, attribution of post 1950 warming to solar activity is tricky (i.e. impossible), because solar activity has declined (slightly) over that time:
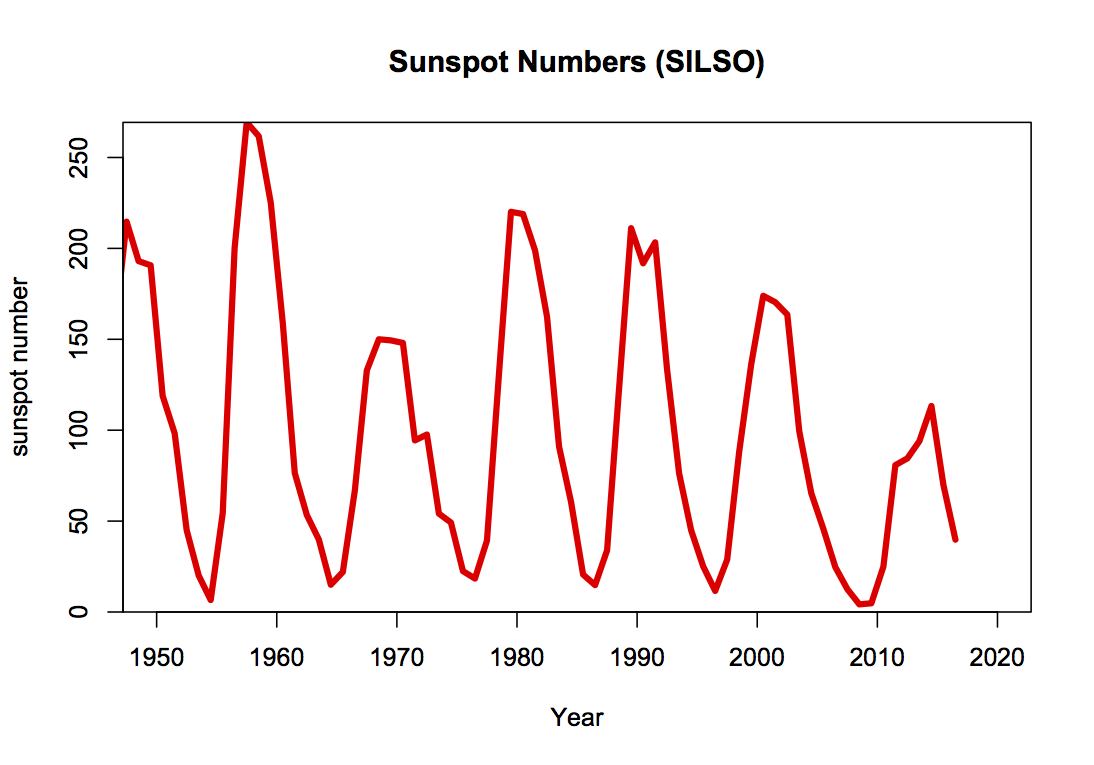
5) Aerosol forcings are indeed uncertain, but this does not impact the attribution of recent trends very much.
One of the trickier issues for fingerprint studies is distinguishing between the patterns from anthropogenic aerosols and greenhouse gases. While the hemispheric asymmetries are slightly larger for aerosols, the overall surface pattern is quite similar to that for greenhouse gases (albeit with a different sign). This is one of the reasons why the most confident statements in IPCC are made with respect to the “Anthropogenic” changes all together since that doesn’t require parsing out the (opposing) factors of GHGs and aerosols. Therefore in a fingerprint study that doesn’t distinguish between aerosols and GHGs, what the exact value of the aerosol forcing right is basically irrelevant. If any specific model is getting it badly wrong, that will simply manifest through a scaling factor very different from 1 without changing the total attribution.
What would it actually take to make a real argument?
As I’ve been asking for almost three years, it is way past time for Curry to shore up her claims in a quantitative way. I doubt that this is actually possible, but if one was to make the attempt these are the kind of things needed:
- Evidence that models underestimate internal variability at ~50-80 yr timescales by a factor of ~5.
- Evidence that indirect solar forcing can increase the long-term impact of solar by a factor of 3 on centennial time-scales or reverse the sign of the forcing on 50-80 yr timescales (one or the other, both would be tricky!).
- Evidence that warm surface ocean oscillations are associated with increased downward net radiation at the TOA. [This is particularly hard because it would mean the climate was fundamentally unstable].
- Evidence that the known fingerprints of different forcings are fundamentally wrong. Say, that CO2 does not cool the stratosphere, or that solar forcing doesn’t warm it.
Absent any evidence to support these statements, the claim that somehow, somewhere the straightforward and predictive mainstream conclusions are fundamentally wrong just isn’t credible.
References
- E.M. Dunne, H. Gordon, A. Kürten, J. Almeida, J. Duplissy, C. Williamson, I.K. Ortega, K.J. Pringle, A. Adamov, U. Baltensperger, P. Barmet, F. Benduhn, F. Bianchi, M. Breitenlechner, A. Clarke, J. Curtius, J. Dommen, N.M. Donahue, S. Ehrhart, R.C. Flagan, A. Franchin, R. Guida, J. Hakala, A. Hansel, M. Heinritzi, T. Jokinen, J. Kangasluoma, J. Kirkby, M. Kulmala, A. Kupc, M.J. Lawler, K. Lehtipalo, V. Makhmutov, G. Mann, S. Mathot, J. Merikanto, P. Miettinen, A. Nenes, A. Onnela, A. Rap, C.L.S. Reddington, F. Riccobono, N.A.D. Richards, M.P. Rissanen, L. Rondo, N. Sarnela, S. Schobesberger, K. Sengupta, M. Simon, M. Sipilä, J.N. Smith, Y. Stozkhov, A. Tomé, J. Tröstl, P.E. Wagner, D. Wimmer, P.M. Winkler, D.R. Worsnop, and K.S. Carslaw, "Global atmospheric particle formation from CERN CLOUD measurements", Science, vol. 354, pp. 1119-1124, 2016. http://dx.doi.org/10.1126/science.aaf2649
@36
There can be no quantitative argument proving that bob is dead or alive. This is because we only know his average breathing rate to even a rough degree of certainty for perhaps the past 50 to “maybe” 100 minutes – an insignificant period given the 100 year duration of his life.
LOL!
I’m coming into the middle of this issue, but have to ask, why is Judith Curry someone who is worth so much attention? Is there a link that explains that?
Tens of Thousands Protest ‘Alternative Facts’ at March for Science
http://www.livescience.com/58792-tens-of-thousands-protest-at-march-for-science.html?utm_source=notification
I was there. I didn’t see you there.
Why not?
37. “No, ice records are not accurate “thermometers”
You are expressing a personal opinion. Accuracy is quantitative term, and not a qualitative one. Accuracy is determined relative to the value being measured, and relative to the accuracy required.
Since you provide no numbers you must be speaking qualitatively which is nothing more than expressing a personal opinion.
And that is why you know so very little.
Gavin,
I don’t see from your histogram of CMIP5 simulations vs. temperature trends how it’s “obvious” that the models are not tuned to the initial data. The histogram mode is visually quite close to the temperature data set means. Maybe I’m wrong, but I don’t think Curry is claiming that the models are a simple curve fitting exercise, which would be required for a high precision fit of the models aggregate to the observed data. And you’re not claiming that the models have poor skill at tracking temperature data in the first place, thus proving that they aren’t tuned, right?
[Response: You can’t tune the ensemble – only individual models. So if individual models were tuned, they would line up far more closely with the observational trend. That the spread is actually much wider is evidence that they weren’t. That the mode of the histogram is quite close is suggests they are (on average) capturing the real forced trend with some variations in internal variability, sensitivity and forcings. – gavin]
Gavin wrote: “Ask yourself one question: Were these models tuned to the observed values?
http://ipcc.ch/report/graphics/images/Assessment%20Reports/AR5%20-%20WG1/SPM/thumbnail/FigSPM-06.jpg
Look at the wonderful agreement between projected and observed warming in Figure SPM-06 from the AR4 SPM. Observations lie right down the middle of projections. Doesn’t look anything like the histogram in this post. The appearance of tuning may have come from CMIP3 models. In those models, high climate sensitivity correlated with increasingly negative aerosol forcing. The same thing was not true for CMIP5. Care to show us the histogram for the CMIP3 models or the data that went into SPM-06?
http://ipcc.ch/report/graphics/images/Assessment%20Reports/AR5%20-%20WG1/SPM/thumbnail/FigSPM-06.jpg
http://ipcc.ch/report/graphics/images/Assessment%20Reports/AR5%20-%20WG1/Technical%20Summary/thumbnail/FigTS-10.jpg
Check out Figure 10-05 to see how the use of scaling factors in fingerprinting reduces the tremendous uncertainty in warming due to GHGs and cooling due to aerosols down to little uncertainty in aligning the combined effects of these forcings with observed warming. As best I can tell, fingerprinting assumes that 100% of warming can be attributed to anthropogenic factors.
When Judith first questioned the attribution statement, she was looking at Figures like these from the IPCC reports, not histogram you included in this post.
Keep Mr. KIA, he’s funny
David @ 20 Apr 2017 at 3:58 PM Regarding the difference between TMT trends in models and satellite measurements, and same sign trend differences between surface temperatures and satellite measurements; I think that inaccurate cloud parameterizations in the models, and resultant inaccuracies in latent and sensible heat transport, including some related to the increases in intense rainfall (the Eno river near where i live has risen from 150 to 5510 CFS in 12 hours). The observed lapse rate under global warming is larger than predicted by models, although the “missing” hotspot has actually been observed – just smaller than models project. Before you (or Dr Christy or Dr. Happer) celebrate too much about this, y’all should consider lapse rate feedback and the implications for climate sensitivity and subsequent impacts.
https://en.wikipedia.org/wiki/Climate_change_feedback#Lapse_rate
Mr Know it all @43 says:
“Scientists today are talking warming in the small range of 0 to 2 C. Paleoclimatology cannot tell us temperature to this degree of accuracy/precision in the distant past. It can tell us that temps went up or down, but there is no way that it can tell us exactly how much.AGW may well be real. The computer models may be correct. But making claims that we know with certainty the global temperature in the distant past is laughable.”
I don’t know who your scientists, are and you give no references.The last IPCC report and climate scientists contributing to the report are talking about warming with business as usual from approx. 3-6 degrees, depending on exact climate sensitivity and levels of CO2 emissions. Good efforts to reduce emissions can bring it down well below this.
Nobody has ever claimed we know past past temperatures with certainty. Studies of the mwp have error bars. However for the mwp we do have reasonably decent temperature re-constructions, and these error bars are approx plus or minus half a degree, not 1 degree or more. You provide no evidence that studies like this are inaccurate as far as they go. Even with error bars like this, its clear we are already warmer than the temperature fluctuations of the mwp, even if the temperatures are right at the outer limit of the error bars. This is significant, although the mwp neither proves or disproves that we are altering the climate, it just gives an indication that we are now altering the climate at a much more rapid pace than the mwp.
SN 53: I was there. I didn’t see you there.
BPL: In my case, that’s because I was at Kent State East Liverpool giving a lecture on global warming and drought.
Don’t assume that because you didn’t see anyone at your party, they must therefore have been idle.
Frank @56,
I can’t see from you links but are the observed and projected warming shown as temperature anomalies? If so there’s your answer.
Eric Swanson, I don’t see how anything you said above is very interesting or of much relevance except to make it clear you are failing to see the forrest for the trees. Real Climate’s graphic shows a large warming rate divergence and 4 datasets total. There is not a big difference between that and Cristy’s graphic which is a simplified version of the same thing with different baselining and averaging methods. It is easy to generate the TMT data from AOGCM’s that includes the small stratospheric component and I believe both Cristy and Real Climate did that.
Scott Nudds @53
I was at the march in Christchurch, New Zealand. I didn’t see you there!
One very minor reply to Gavin@1:
‘For some reason Judy thinks that I think that “most”, “>50%” and “more than half” are distinct quantities. For the record, I do not.’
For the record, I do, and many others do. For me, ‘most’ means ‘a large majority’, where ‘large’ is rather vague; I’d say more than 70%, though. I was surprised to discover about twenty years ago that others took it to be >50%; when I asked educated acquaintances I found that both interpretations were common but (almost?) nobody was aware of the one different from their own. This is not a matter of arithmetical incompetence but simply different word usage. Apparently most–er, a large majority of–uses of ‘most’ allow both interpretations. I don’t recall another example since that initial discovery.
None of which is meant to deny that Curry is disingenuous, ignorant, a liar, or all of the above.
Also, ‘the most’ is a different story.
Brian Dodge said:
I know Mann has said that the absence of the hotspot would imply higher ECS. But there is strong evidence that this is wrong. Nic Lewis points out the following.
Hyperacitve Hydrologist say: “I can’t see from you links but are the observed and projected warming shown as temperature anomalies? If so there’s your answer.”
I tried to paste links to the figures themselves, which would insert them into comments at other blogs. That approach didn’t work here.
Since the Figure are both from the IPCC, they (of course) refer to temperature anomalies. Anomalies are the most practical way to deal with CHANGE in temperature. (And they hide the fact that many AOGCMs don’t reproduce current absolute GMST very accurately.) Anomalies don’t provide a useful answer.
The first link was supposed to point to Figure SPM.4 from AR4 WG1. It shows that observed warming tracked perfectly with the mid-point of warming hindcast by CMIP3 models. This was the data FIRST using by the IPCC to claim with extraordinary confidence that at least 50% of warming was caused by man and started Judith Curry down the path towards skepticism. Unfortunately, I linked the corresponding figure from AR5 (SPM.6). Neither figure looks anything like the histogram in this post. IMO, Gavin’s histogram shows that the average climate model is running hotter than observations, a problem that would be even worse if he he included troposphere warming derived from MSU data! You’ll have to ask Gavin why these figures from the IPCC look so different from the histogram he posted. (I suspect part of the explanation depends on the difference between CMIP 5 and CMIP 3 models. The latter showed a strong correlation between high ECS and high sensitivity to aerosols and probably agreed more closely with observations. In any case, Judith deserves to be judged by the evidence the IPCC published, not the histogram presented in this post.
https://www.ipcc.ch/pdf/assessment-report/ar4/wg1/ar4-wg1-spm.pdf
https://www.ipcc.ch/pdf/assessment-report/ar5/wg1/WGIAR5_SPM_brochure_en.pdf
Since attribution studies (fingerprinting) use scaling factors to adjust for the spread in hindcast warming, those studies create the impression of close agreement between hindcast and observed warming. The second link was intended to point to Figure 10.5 of AR5, Chapter 10, page 884. Notice that, after scaling, “anthropogenic warming (the orange bar) and observed warming (the black bar) are in close agreement with NARROW confidence intervals, despite the massive uncertainty in how much warming was attributed to GHGs (green) and cooling to aerosols (yellow). IMO, it is perfectly sensible to be skeptical about the value of a process that converts the large uncertainty in Gavin’s histogram and in the green and yellow bars into close agreement between observed and hindcast warming.
(Perhaps this represents ignorance on my part. However: all causes of warming produce amplification at high latitudes. CFCs are cooling the stratosphere. If MSU temperatures were used in place of surface temperature), what would fingerprinting show?)
Different parameterizations of one model can exhibit different climate sensitivity and still represent current climate equally well. A recent publication from the GFDL group shows that ECS drops 1 K (with no decrease in accuracy at representing current climate) when the parameters controlling convection were moved between two of their IPCC models. An excerpt from Section 10.1 of AR4 (p 753) discusses the parameterization problem:
“Many of the figures in Chapter 10 are based on the mean and spread of the multi-model ensemble of comprehensive AOGCMs… Since the ensemble is strictly an ‘ensemble of opportunity’, without sampling protocol, the spread of models does not necessarily span the full possible range of uncertainty, and a statistical interpretation of the model spread is therefore problematic.”
http://www.ipcc.ch/pdf/assessment-report/ar5/wg1/WG1AR5_Chapter10_FINAL.pdf
In other words, there is no rigorous process for deriving probabilities from the IPCC’s “ensemble of opportunity”. Such conclusions represent “expert judgment”. Judith Curry is certainly qualified to express her expert judgment.
Finally. Gavin wrote: “Models are NOT tuned [for the late 20th C/21st C warming] and using them for attribution is NOT circular reasoning”
However, Isaac Held discusses at his blog how climate models can be indirectly tuned agree with observed warming and concludes: “I think it’s reasonable to assume that there has been some tuning, implicit if not explicit, in models that fit the GMT evolution well.” (The slowest warming out in the histogram comes from one of their three models.) In 1991, Lorenz clearly warned about circular reasoning in the conclusion of a prophetic paper: “This somewhat orthodox procedure [attribution using AOGCMs] would be quite unacceptable if the new null hypothesis had been formulated after the fact, that is, if the observed climatic trend had directly or indirectly affected the statement of the hypothesis. This would be the case, for example, if the models had been tuned to fit the observed course of the climate.” No one likes Judith Curry for pointing this out.
https://www.gfdl.noaa.gov/blog_held/73-tuning-to-the-global-mean-temperature-record/#more-43597
E.N. Lorenz (1991) Chaos, spontaneous climatic variations and detection of the greenhouse effect. Greenhouse-Gas-Induced Climatic Change: A Critical Appraisal of Simulations and Observations, M. E. Schlesinger, Ed. Elsevier Science Publishers B. V., Amsterdam, pp. 445-453. http://www.iaea.org/inis/collection/NCLCollectionStore/_Public/24/049/24049764.pdf?r=1
At Climate Etc., I have expressed the opinion that focusing on the attribution issue is counter-productive. By assuming that essentially all warming is due to man, energy balance models produce a best estimate for ECS between 1.5 and 2.0 K. ECS is certainly is not below 1 K, so a BEST ESTIMATE is that more than 50% of warming certainly must be anthropogenic (despite evidence from past climate change showing that unforced or natural warming could be responsible). The real problem is that many AOGCMs exhibit an ECS that is twice as high as EBMs. This problem is clearly illustrated (for TCR) by the difference between observed and hindcast warming in this post.
David #62 – The Real Climate graph in this post compares the model results with the surface temperature results and the surface data appears to be in the middle of the model spread. May I remind you, we live at the surface, not the middle troposphere.
Christy’s graph for the US House Science Committee suggests that there’s a large difference between the model results and the satellite “Mid Troposphere” product. In his testimony, Christy never mentions the fact that the MT must be corrected to compensate for the stratospheric influence and the graph leaves the unaware with the impression that there is a large difference between the model results and the measurements. And, your claim that “It is easy to generate the TMT data from AOGCM’s…” displays a lack of understanding of exactly what’s involved with such a transformation. There’s no documentation from Christy to indicate what he did, such as: the weighting of different pressure levels, simulating the influence of hydrometeors from storms, the emissions from various surface types over the annual cycle from the model results and the impact of high elevations, such as over the Antarctic. If you’ve got a reference, let us have it.
What you assert or happen to believe is not factual evidence. Blog posts don’t count in science where peer review is essential. Better luck next time…
Of course, there is one error in the OP, indeed in the opening paragraph of the OP.
It states Judy
This is untrue. Judy’s response does contain “quantitative argument.”
Judy describes the attribution of the last century’s warming to AGW as being about “HOW MUCH” and says:-
“Two scientists!” That sounds qunatitative to me.
Of course, this does not mean the “quantitative argument” has any merit. Indeed, where are the error bars on this data? As this is Judy & John, the errors will be humogously massive, so two, twenty, two-hundred: at such levels the data is scientifically insignificant.
Eric wrote @67: “In his testimony, Christy never mentions the fact that the MT must be corrected to compensate for the stratospheric influence and the graph leaves the unaware with the impression that there is a large difference between the model results and the measurements.”
This is totally incorrect. He said that same methodology was used to process both observations and models when separating changes in the stratosphere from changes in the troposphere. If the UAH troposphere record is contaminated with stratospheric cooling, then so are the models.
(Your argument might explain why the troposphere hasn’t warmed as much as the surface, but it can’t explain the difference between models and observations.)
Frank #69 – Here’s a quote from Christy’s written testimony on 29 March:
“In this testimony I shall focus on the temperature of the bulk atmospheric layer from the surface to about 50,000 ft. – a layer which is often called by its microwave profile name TMT (Temperature of Mid-Troposphere).”
Christy then proceeds to present a plot of results from the Canadian climate model, which indicates an expected warming above the tropical region and outlines the area which he says is represented by the TMT. The plot does not indicate that the TMT also receives emissions from higher up into the Stratosphere and nowhere is there any indication that the graph is adjusted to represent simulated TMT measurements.
Christy then writes:
“The first type of observational datatset is built from satellites that directly measure the bulk atmospheric temperature through the intensity of microwave emissions.”
Again, there’s no mention of the stratospheric component, which causes the TMT to exhibit a lower trend. Further on, Christy writes:
“The second type of measurement is produced from the ascent of balloons which carry various instruments including thermistors (which monitor the air temperature) as the balloon rises through this layer. From these measurements a value equivalent to the satellite TMT profile is calculated.”
Christy has added Reanalysis data and describes these data thus:
“From the information at the vertical levels the TMT quantity is generated for an apples-to-apples comparison with models, satellites and balloons.
Christy continues, writing:
“In Figure 2 we show the evolution of the tropical TMT temperature since 1979 for the 102 climate model runs grouped in 32 curves by institution….The curves show the temperature evolution of the atmosphere in the tropical box shown in Fig. 1.”
The problem I have with this is the insinuation that the TMT actually represents the temperature history of the troposphere, (which it clearly does not) and that his analysis applies to the modeled trends in Figure 1. Nowhere does Christy describe the process to create the various simulated TMT data and there’s no reference material to consult. In a blog post, Spencer gave some numbers for the weighting values applied at different pressure levels, but there’s no discussion of how these weights were derived and whether they were applied to all latitudes and for all seasons. Of course, the model results are zonally averaged, so it’s impossible to distinguish local effects, such as ocean vs. land, high elevations and any changes in storm intensity producing hydrometeors. In other words, Christy’s results are themselves based on a model, which is not discussed but which is at the core of his comparisons.
Frank @69
Eric’s explanation that the Mid troposphere satellite temperatures are being contaminated with stratosphere temperatures does not explain why the radiosondes agree with the MSUs. In other words, the models do not match the radiosonde measurements. Why the MSUs and models disagree has been an open question for 20 years now. How much longer is it going to take for the models to be investigate with the same intensity as the satellite data. Actually the answer is in my poster from EGU 2016 here: A Paradigm shift to an Old Scheme for Outgoing Longwave Radiation.
“If the UAH troposphere record is contaminated with stratospheric cooling, then so are the models.”
Not.
Even.
Wrong.
How, pray tell, would model MT temperatures be “contaminated” by stratospheric cooling? The models don’t determine the modelled MT temperature by modelling microwave emissions from oxygen as seen through the stratosphere, they calculate the temperature directly.
Frank @69,
You tell us of Christy’s testimony:-
I think you need to be showing what was actually “said” by Christy. His testimony is only a dozen pages but I cannot see what you are referring to. Perhaps it is a different document you are introducing into the discussion.
Just on this issue with UAH satellite data on temperature trends in the middle of the atmosphere showing a little less warming than surface temperatures like Nasa giss, particularly since about 2000. The standard explanation are satellites are inaccurate etc.
However could this difference relate to the fact that the surface is warming partly due to positive feedbacks in the arctic, and this is enough to significantly influence the global average and push it up? this sort of surface warming in the arctic is regional, and would stay near the surface at least for some time.
Eric Swanson, This is really very simple. Aside from “messaging” which seems to be your concern, the science is clear and consistent.
1. Christy and Real Climate both show that there is a big divergence between measured TMT and Aogcm modeled TMT.
2. Christy said in his testimony that TMT was done consistently and the stratospheric component was included correctly. Real Climate did it correctly too.
3. The hot spot issue is important as my comment #65 shows. There is probably a problem with convection, cloud, precipitation models.
Christy could show Gmst as well . This is not as convincing either as some would like to believe. I guess my question is have you looked at the Real Climate figure and do you dispute it?
I have found that models which look good when run over the period where data is available may reveal their true colors when run over other periods. Just run the models you most believe in backwards for a century or so, without any changes except those necessary to make them run backwards. Tell us what you find without any modifications. My bet is that they will all reveal themselves to be unstable, divergent, or exhibiting other nonsensical behaviors, which will serve as clues to where they are unrealistic. We have some elementary records for the last few centuries to rule out the existence of extreme results.
[Response:
– gavin]
Thanks very much for the response. You have corroborated my worries with the image and the reference. The chapter on model evaluation does not seem to have one sentence on ‘backcasting’, which is a much more severe test than the others listed, as it takes the model out of its comfort space of known data, where tuning is king. I would be nervous making any conclusions from these models, but more brave souls might. Here is my antique reference to it: http://ieeexplore.ieee.org/stamp/stamp.jsp?arnumber=4309570 , googling Erickson/Pikul or backcasting will give you some other comments on the technique.
[Response: Try actually talking to someone who knows what they are talking about. The term used in climate modeling is “hindcasting” (not ‘backcasting’) and so searching on the wrong word isn’t going to be informative. All of the runs in the figure I posted are hindcasts – exactly what you asked for. The forecasts from the same models are viewable on the model/obs comparison page. – gavin]
Here’s something on it: http://www.sjsu.edu/faculty/watkins/backcasting.htm
[Response: This is nonsense written by someone who does not know anything about climate models. Try our FAQs for more accurate info. – gavin]
StanFL – you might be have been right if the GCMs were statistical models but they are not. They are physical models. Models for say rocket trajectory work as well around Jupiter as around Earth for the same reason.
Further to above, StanFl, GCMs are used to investigate old climates like the last ice termination. (eg https://hal.archives-ouvertes.fr/file/index/docid/298083/filename/cp-3-423-2007.pdf). More interesting question, dont you think it a bit rich to be assuming experts wrong and using models badly when you obviously havent even bothered to read the papers?
Shoulda let StanFL put up some money for his bet, before showing him he’s lost it.
Since there are essentially no controlled experiments for climate science, all we can do is do hindcast analyses by trying to hide training intervals from out-of-band testing intervals. This is tricky because the more data that is used, the better one can resolve the mechanism; yet applying all of the data can also lead to potential over-fitting without having access to pristine test intervals.
It’s saint versus taint — be a saint and close your eyes to pristine data or be a taint and use it all.
As a layman who seriously tries to understand the state of the climate debate in a neutral, objective way, I think the most revealing aspect of this is the silence from Dr. Curry.
If I were to see someone give a substantial criticism of my views, such as this post, I would make it my first priority to understand the criticism, its strengths and weaknesses, and then publish an appropriate response. A cycle of public debate and scientific work would emerge, propelling the debate forward and enlightening everyone. Unfortunately, that has not happened because Dr. Curry refuses to engage.
We should all pause to ask ourselves why this is the case, and why so frequently the debate seems to suddenly end with the ball in the climate skeptic’s court.
It should be very suspicious, even to the casual layman reader, when one side claims some kind of epistemic high ground but refuses to defend that ground in substantial debate. It should be even more suspicious when this same person instead prioritizes putting herself forth as an “expert witness” to policyholders.
#83 Curry is doing what her minders want her to do for years. There’s no basis to assume she’s honestly mistaken. There’s a lot of money to be made in private climate weather predictions. Marohasy et al are on the same neoliberal funded gravy train.